Case study: RlapsRisk® BC
Authors
More like this
Prediction of patient prognosis from digitized pathology slides in early Breast Cancer (ER+/HER2-)
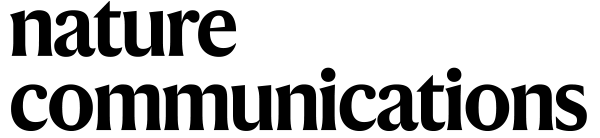
Context
Breast cancer (BC) remains the leading cause of cancer death for women worldwide, despite therapeutic advances. Understanding a patient's likelihood of reoccurrence is critical to determine their treatment strategy. However, assessing this risk is not always straightforward due to the heterogeneity of disease and range of subtypes associated to disease prognosis.
Methods
Owkin developed RlapsRisk BC, an AI-driven solution that assesses the risk of distant relapse at 5 years of ER+/HER2- early invasive (ei)BC patients, post surgery, from H&E (hematoxylin-eosin)-stained whole slide images (WSI) and clinical data.
The solution included 1800+ patients in its training cohort and was validated on independent cohorts of 1200+ slides, in a blind single shot fashion.
Results1
Using digital pathology slides, this model accurately discriminates between low and high risk breast cancer patients (ER+/HER2) through independently predicting Metastasis Free Survival (MFS).
Combining RlapsRisk BC AI score and the clinico-pathological factors improved the prognostic discrimination (c-index 0.81) compared to the clinico-pathological factors alone (c-index 0.76). Significantly, RlapsRisk BC can provide an additional layer of information to better define the risk of relapse - even in subgroups where the risk of relapse is particularly difficult to assess.
Impact
Diagnostic:
- Help oncologists and pathologists assess the risk of relapse of eBC patients
Optimize clinical development:
- Stratification biomarker to select high value subgroups, improving RCT statistical power
- Inform on the most prognostic variables to increase RCT statistical power
Testimonial
RlapsRisk® BC can predict the risk of recurrence for ER+/HER2- breast cancer patients at the time of diagnosis. Professor Catherine Guettier, former Head of Pathology at Hopital Bicètre Greater Paris University Hospitals - AP-HP, showcases how they’re using digitized slides and CaloPix®, Tribun Health's IMS system, to support pathologists and oncologists in the future, making more informed treatment decisions and improving patient outcomes.
1. Garberis, I., Gaury, V., Saillard, C. et al. Deep learning assessment of metastatic relapse risk from digitized breast cancer histological slides. Nat Commun16, 5876 (2025). https://doi.org/10.1038/s41467-025-60824-z
Learn more about RlapsRisk BC
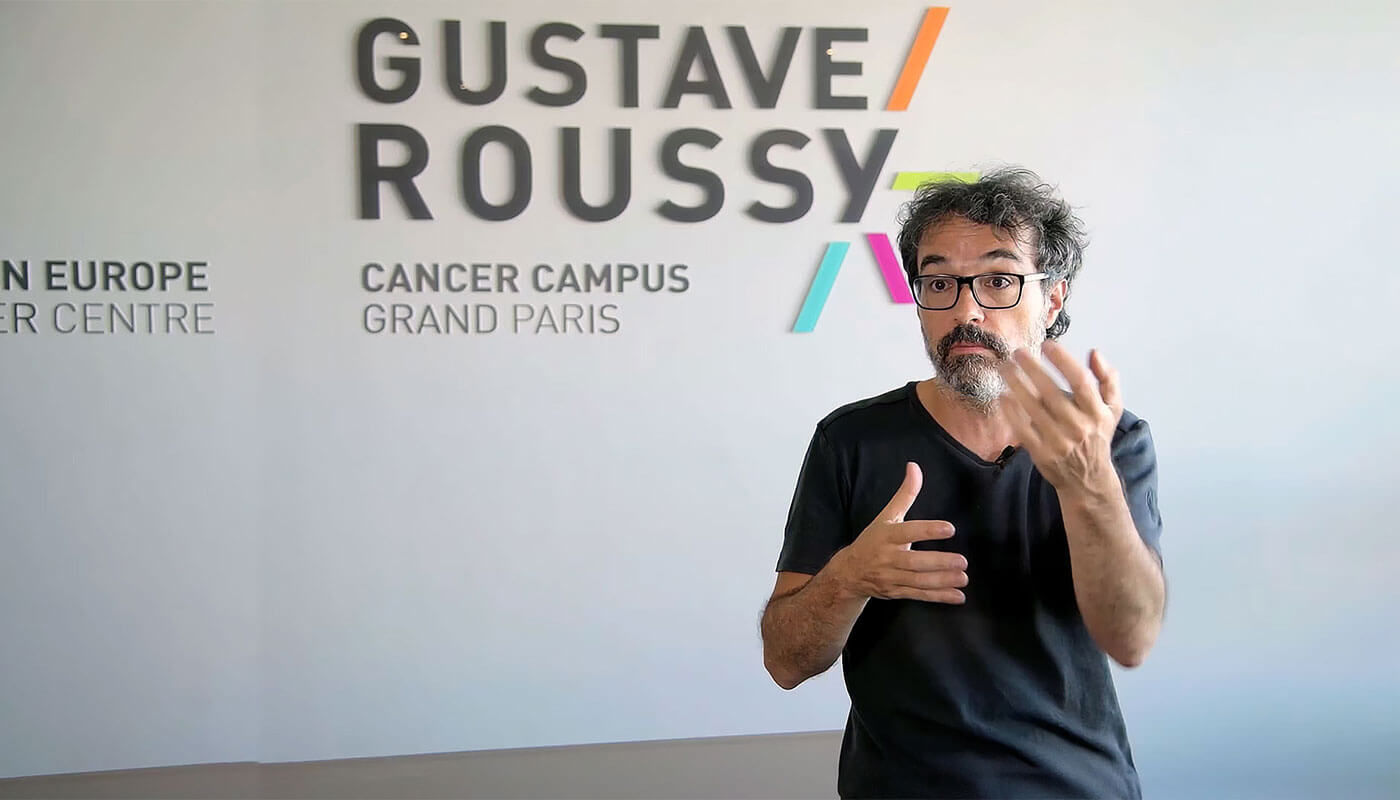