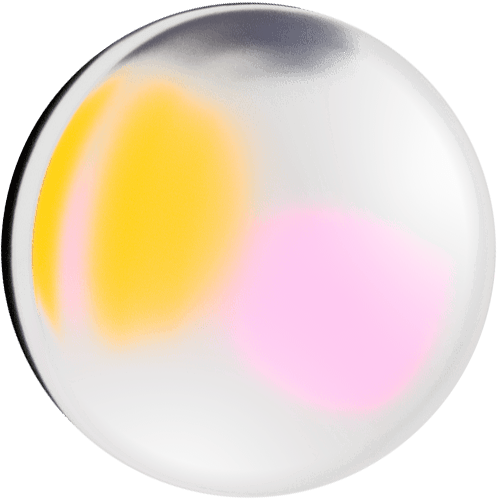
RlapsRisk® BC
Assess the risk of breast cancer relapse
Clinical context
Revolutionize breast cancer care management by democratizing access to actionable medical insights
Clinicians lack the tools to identify these high-risk patients at an early stage. Current methods either lack consistency in accuracy 4,5,6 or they are expensive and non-routine, 7 such as genomic sequencing.
Therapeutic and pathologist workflow
Integrating AI-powered diagnostics seamlessly
Subtype confirmed
ER+/HER2- invasive carcinoma of the breast
Surgery
Tumor excision
Pathologist
Selects slide of indication
WSI Review
Analysis of digitized slide
Owkin diagnostic
RlapsRisk BC
PDF report
Pathologist
Delivers final pathology report
Multidisciplinary board
Further testing / treatment decision
Incoming President of ESMO
Research and development
Timeline of milestones
Time-dependent AUC
Clinical score: 0.772
AI model: 0.77
AI model integrating clinical score: 0.822
Image AI analysis outperforms clinical scores
RlapsRisk® BC combined with a clinical score composed of standard clinicopathological criteria achieves 76% cumulative sensitivity and 76% dynamic specificity at 5 years. 1
In comparison, the clinical score alone achieves a cumulative sensitivity of 61%, for the same specificity. RlapsRisk® BC can identify 15% more patients who will likely relapse through classifying them as high risk at the time of diagnosis. 1
Cumulative Sensitivity/Dynamic Specificity are natural extensions of sensitivity/specificity to the setting of time-to-event outcomes, such as the metastasis-free survival (MFS), understood as the time to distant relapse occurrence from initial surgery. In use here, as they easily accommodate time-dependent outcome status as well as right-censoring.
The clinical score is composed of the following variables: histological grade, age at diagnostic, tumor size, lymph nodes invasion, and Ki67 expression.
Citations
- Manuscript under review with peer reviewed journal, and available as a pre-print on bioRxiv: https://www.biorxiv.org/content/ 10.1101/2022.11.28.518158v2.full
Performances are combined score of clinical scores and RlapsRIsk® BC. - https://www.bcrf.org/breast-cancer-statistics-and-resources/
- Long-term hazard of recurrence in HER2+ breast cancer patients untreated with anti-HER2 therapy, Strasser-Weippl et al. 2015, BMC
- Gown AM. Current issues in ER and HER2 testing by IHC in breast cancer. Mod Pathol. 2008 May;21 Suppl 2:S8-S15. doi: 10.1038/modpathol.2008.34. PMID: 18437174.
- Casterá C, Bernet L. HER2 immunohistochemistry inter-observer reproducibility in 205 cases of invasive breast carcinoma additionally tested by ISH. Ann Diagn Pathol. 2020 Apr;45:151451. doi: 10.1016/j.anndiagpath.2019.151451. Epub 2019 Dec 17. PMID: 31955049.
- Polley MY, Leung SC, McShane LM, et al. An international Ki67 reproducibility study. J Natl Cancer Inst. 2013 Dec 18;105(24):1897-906. doi: 10.1093/jnci/djt306.
- Blok EJ, Bastiaannet E, van den Hout WB, et al. Systematic review of the clinical and economic value of gene expression profiles for invasive early breast cancer available in Europe. Cancer Treat Rev. 2018 Jan;62:74-90. doi: 10.1016/j.ctrv.2017.10.012.