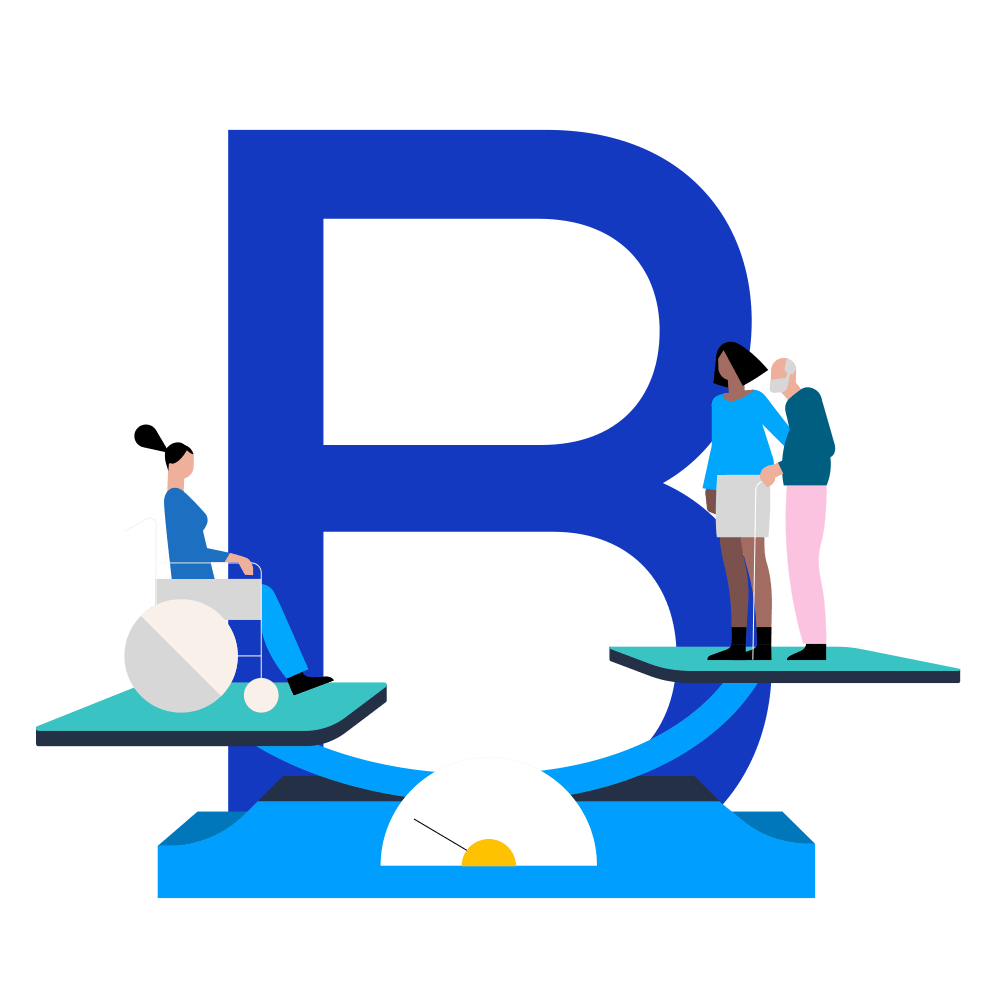
Bias
The dictionary definition of bias is ‘any tendency to support or oppose a particular person (or group of people) or thing in an unfair way because of personal opinion.’ This can sometimes be positive, helpful, or at least not harmful. For example, it is reasonable to be biased toward well-lit streets at night and biased against highly-processed foods.
However, the biases that people form towards other people are often based on unjustified stereotypes associated with characteristics such as race, ethnicity, gender, religion, sexual orientation, socioeconomic background, or educational attainment. In a hospital setting, these biases can result in people of specific genders, sexual orientations, or ethnicities sometimes receiving inaccurate diagnoses and or poorer quality treatment.
Bias can also appear, or occur, in medical research. For example, if the people involved in a drug trial (the ‘sample’) largely consist of white men in their twenties, then there is likely to be a deviation (i.e. a bias) between the research results and the ‘facts’ of reality when the findings are applied in real life settings across more diverse populations.
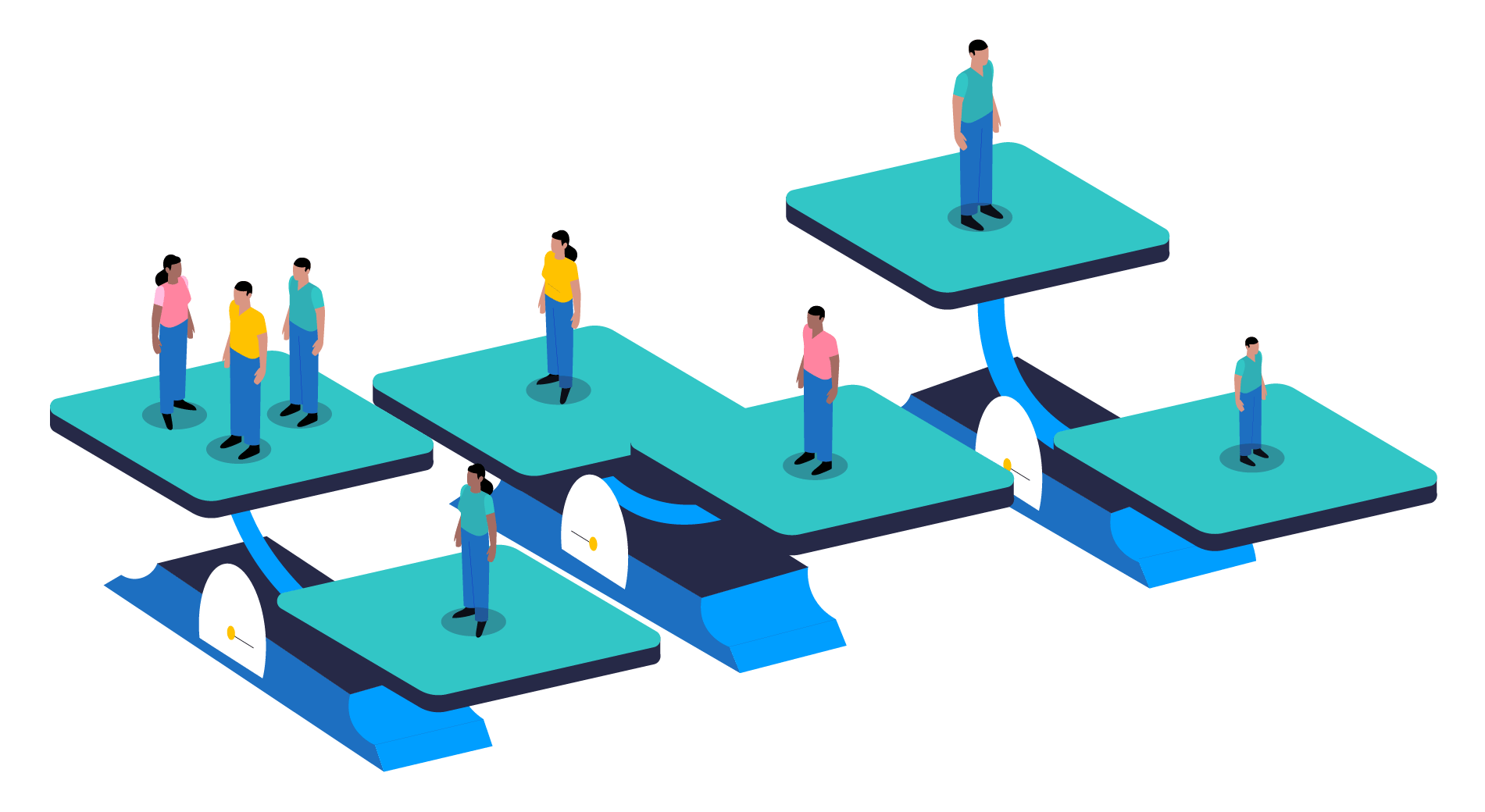
These biases can be problematic. For example, if a drug has been tested on a ‘sample’ in which women or people of colour are under-represented then it might not work as well for these individuals when it is prescribed to them – indeed, it might even cause them harm.
The existence of these and other types of bias matter in the context of artificial intelligence because they’ve become embedded in the datasets that AI algorithms are trained upon. For instance, there is evidence that algorithms embedded in smartwatches for the purpose of detecting ‘atrial fibrillation’ (irregular heartbeat) work less well for users with darker skin than lighter skin – most likely due to the overrepresentation of lighter skin patients in the training datasets.
Such biased algorithms may provide a poorer standard of care to the patients against which they are biased. Fortunately, there are steps that can be taken to adjust for bias and its consequences. For example, algorithms trained on more diverse population data, tested and validated independently, and monitored once in use are far less likely to be biased.
There are at least three different ways in which bias can arise in a healthcare context: clinical research, clinical consultation, and clinical statistics.
To start with clinical research, bias in this context is a ‘systematic (i.e. reproducible and not random) distortion of the relationship between a treatment, risk factor, and clinical outcome.’ It can occur in the planning, data collection, analysis, and publication phases of research and there are multiple different categories including selection bias, information bias, reporting bias, and confounding.
All types of research bias, regardless of their source, can result in false conclusions being drawn from the research, loss of external validity, loss of generalisability, and potentially harm to patients. Perhaps the most well-known incidence of bias of this type is the retracted 1990s study falsely linking the MMR vaccine to autism, which was later shown to be biased in several ways including a very small (and non-representative) sample size, and over-inflation of results.
Next, when the word bias is used in the context of a clinical consultation, typically what is being described is ‘cognitive bias.’ Cognitive bias is the tendency for humans to rely on ‘intuitive’ or ‘fast thinking’ rather than slower analytical thinking. Intuitive thinking relies heavily on heuristics and mental models that enable quicker decision-making, by reaching conclusions based on just a few factors. For example, deciding that a woman presenting in a clinic complaining of weight-gain, nausea, and tiredness is most likely pregnant. This is often a largely autonomous process and is typically based on ‘implicit’ or ‘unconscious’ bias (i.e. bias that the clinician is not aware of). Problems arise when the cognitive biases relied upon by clinicians to make rapid decisions are based on harmful and unjustified stereotypes associated with characteristics such as race, ethnicity, gender, sexual orientation, geographic location, educational attainment, or socioeconomic status. Negative bias of this kind can result in diagnostic errors and poorer outcomes for patients.
Another type of non-systemic bias is automation bias. Automation bias is where humans assume that computers are always right and so take any information provided by a computer at face value. Clinicians and those engaged with AI should feel comfortable and confident enough to review the outputs of any AI algorithm used in their practice and to question it if they do not think it looks correct or if it feels ‘off.’
Finally, in a statistical sense, bias is anything that leads to a systematic difference between the true parameters of a population and the statistics used to estimate those parameters. Essentially, it is anything that results in a systematic difference between the ‘results’ and ‘the truth.’ Most often this type of statistical bias arises from incomplete or unbalanced (i.e. biased) datasets used to train statistical models (including Artificial Intelligence or Machine Learning algorithms).
These datasets may be biased due to cognitive biases in the consultation, leading to biased clinical coding of electronic health record data (for example, overdiagnosis of one condition in one group of people and underdiagnosis of it in another group of people), or bias in clinical research, both resulting in the creation of biased and non-representative datasets. Resultant biased ‘algorithms’ when used in healthcare settings, may result in uneven distribution of resources, unwarranted variations in care, and ultimately discrimination.
None of these sources and types of bias can be eradicated, nor can bias be measured in a binary sense i.e., present or absent, most likely all medical algorithms will be biased to a greater or lesser extent, just as all clinical research is biased to a greater or lesser extent. What is possible, however, is the mitigation and reduction of bias.
Furthermore, the ‘black box’ nature of some medical algorithms means that the source of bias can sometimes be difficult to identify and correct. For these reasons, and others, all those responsible for developing, deploying, and using AI algorithms for healthcare should be aware of the risk of bias.
An Owkin example
In September 2022, Owkin and 14 research partners launched an NIH-funded project titled Voice as a Biomarker of Health, with the aim of building a diverse dataset that can be used to train machine learning models to spot diseases by detecting changes in the human voice. The development of large diverse datasets is one of most effective means of mitigating bias in AI algorithms.
The challenge is that whilst there are well-known strategies for mitigating human cognitive biases and bias in clinical research, such strategies are not yet well-known or widespread in the context of Artificial Intelligence, which also introduces the risk of ‘latent bias’ (i.e., bias that develops overtime as a result of population or dataset drift).
Further reading
- Challen, Robert et al. 2019. ‘Artificial Intelligence, Bias and Clinical Safety’. BMJ Quality & Safety 28(3): 231–37.
- DeCamp, Matthew, and Charlotta Lindvall. 2020. ‘Latent Bias and the Implementation of Artificial Intelligence in Medicine’. Journal of the American Medical Informatics Association 27(12): 2020–23.
- FitzGerald, Chloë, and Samia Hurst. 2017. ‘Implicit Bias in Healthcare Professionals: A Systematic Review’. BMC Medical Ethics 18(1): 19.
- Gopal, Dipesh P et al. 2021. ‘Implicit Bias in Healthcare: Clinical Practice, Research and Decision Making’. Future Healthcare Journal 8(1): 40–48.
- Hammond, M. Elizabeth H., Josef Stehlik, Stavros G. Drakos, and Abdallah G. Kfoury. 2021. ‘Bias in Medicine’. JACC: Basic to Translational Science 6(1): 78–85.
- M Delgado-Rodríguez and J Llorca. 2004. ‘Bias’. Journal of Epidemiology and Community Health 58(8): 635.
- Noor, Poppy. 2020. ‘Can We Trust AI Not to Further Embed Racial Bias and Prejudice?’ BMJ: m363.
- O’Sullivan, Ed, and Sj Schofield. 2018. ‘Cognitive Bias in Clinical Medicine’. Journal of the Royal College of Physicians of Edinburgh 48(3): 225–32.
- Pannucci, Christopher J., and Edwin G. Wilkins. 2010. ‘Identifying and Avoiding Bias in Research’. Plastic and reconstructive surgery 126(2): 619–25.
- Šimundić, Ana-Maria. 2013. ‘Bias in Research’. Biochem Med 23(1): 12–15.
- Smith, Joanna, and Helen Noble. 2014. ‘Bias in Research: Table 1’. Evidence Based Nursing 17(4): 100–101.