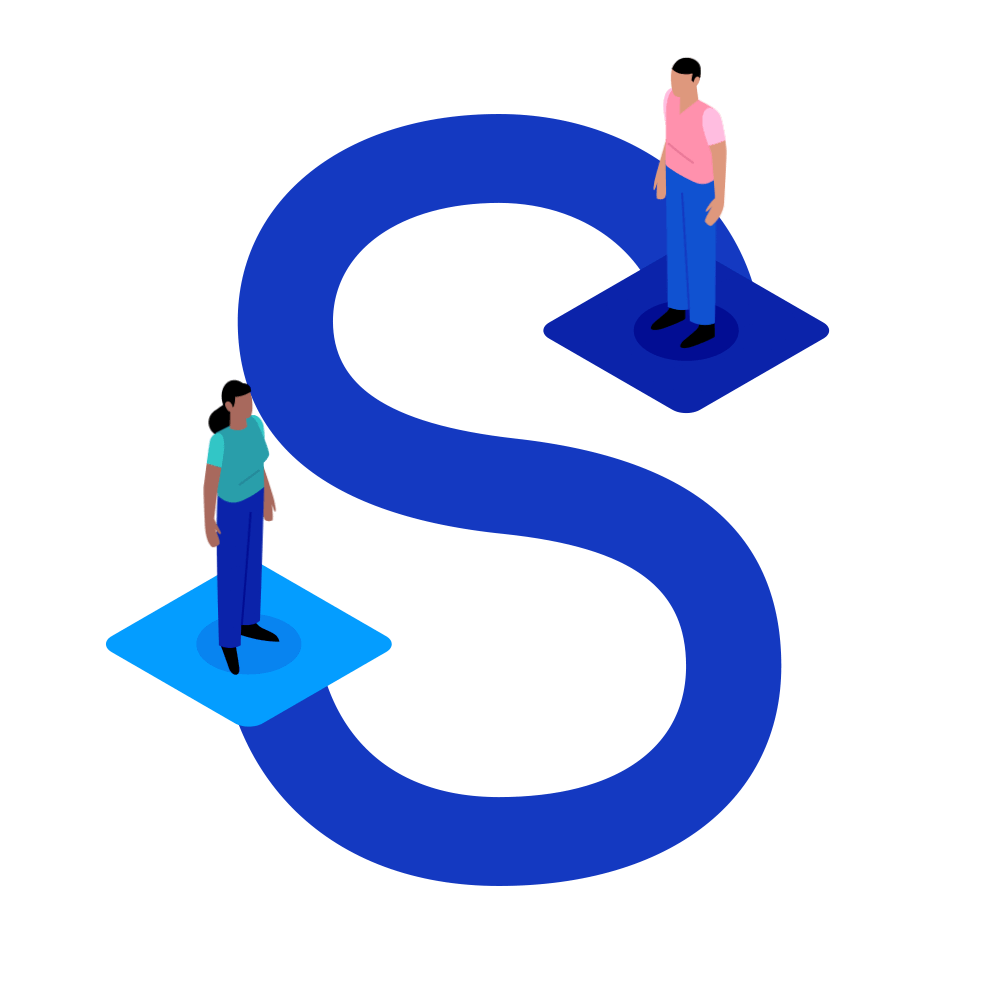
Subgroup determination
Drugs often have different effects on different groups of people, both in terms of how the drug affects the patient and the size of that effect. These different groups are known as ‘subgroups’ of the population.
When developing a new drug or new treatment, it’s important to understand which patients respond best to the drug (i.e., have the best results), which patients have a moderate response (i.e., have OK results), and which patients have a negative response (i.e., have bad or dangerous results such as an allergic reaction). These three types of patients are known as ‘subgroups’ because they are smaller groups within an overall population.
Working out which patients belong in which group is a process known as subgroup determination.
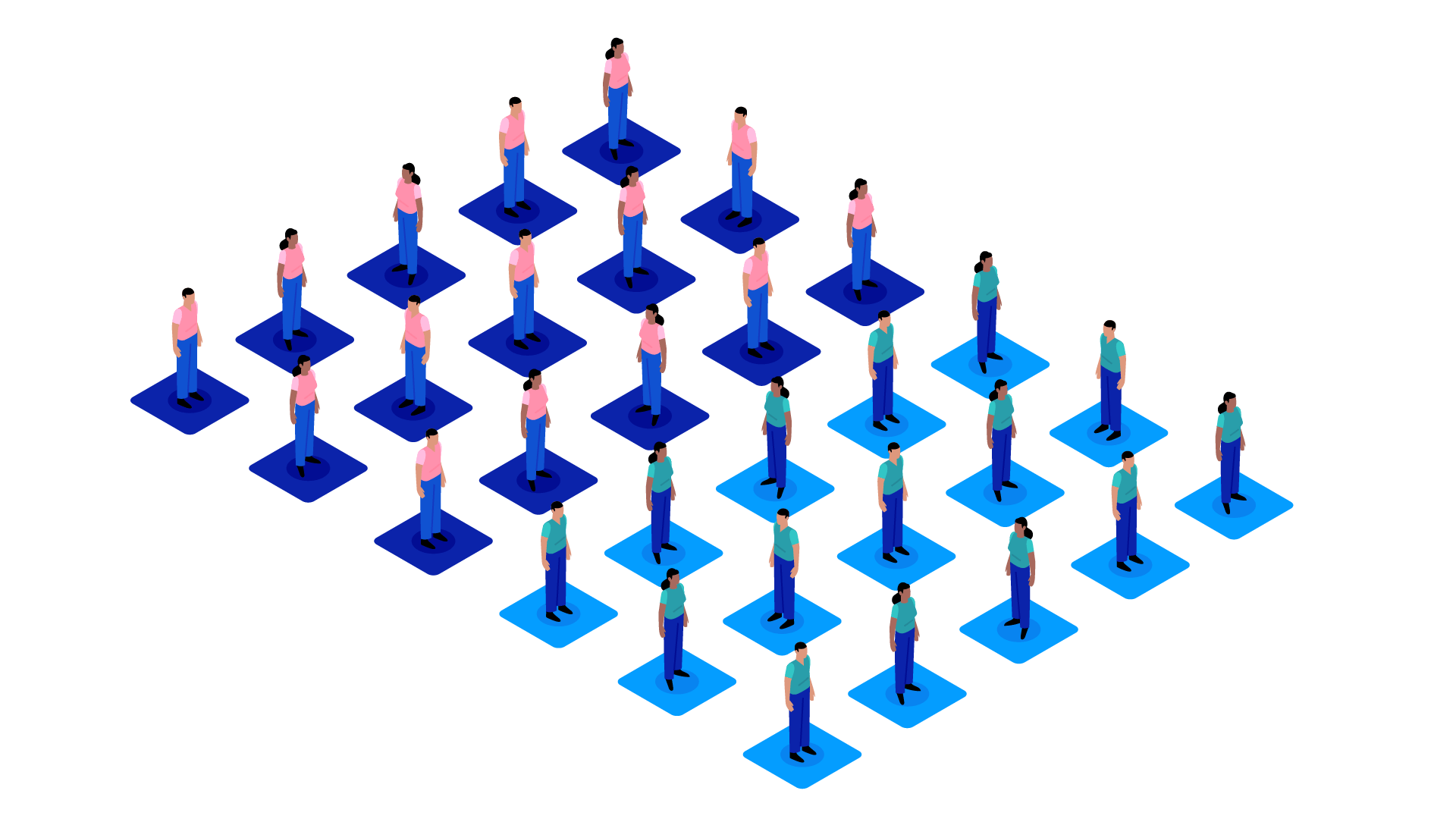
AI, particularly unsupervised AI, can help with this process. For example, you might give an unsupervised algorithm an unlabelled dataset containing the electronic health records of all the patients enrolled in a clinical trial including the ‘results’ of the new drug or treatment (e.g., lowering blood pressure). You can then tell the algorithm to group similar patients according to the results (a task known as clustering). In this way, the algorithm can determine the subgroups and assign patients into the different groups.
Using AI to help with subgroup determination like this can be helpful because it might indirectly identify potential ‘causes’ for the different responses amongst patients that were not immediately obvious. It might, for example, identify that all patients that were in the ‘good response’ group were between the ages of 18 and 45, all those with a moderate response were between the ages of 45 and 65, and all those with a poor response were over the age of 65.
This is very useful data because if the drug was to ever be made available to clinicians as a possible treatment for blood pressure, this information might contribute towards possible guidance issued recommending that the drug be used as the primary treatment for younger patients with high blood pressure, but not as the primary treatment for older patients.
Drugs often have different effects on different groups of people, both in terms of how the drug affects the patient and the size of that effect. These different groups are known as ‘subgroups’ of the population.
Identifying or determining these subgroups and the variations in their treatment response is a crucial step in drug discovery, drug safety monitoring, and the development of personalised medicine. This can be done both prospectively (i.e., ahead of a clinical trial) and post-hoc (i.e., after the trial has started and during the real-world testing of the drug).
Prospective subgroup identification (known formally as confirmatory subgroup analysis) involves the identification of a small number of predefined covariates (typically demographic patient characteristics known as biomarkers) that are listed in the registered trial protocol. For example, the COVID-19 vaccine trials, recruited trial participants and tested the various COVID-19 vaccines, in different age groups.
Post-hoc or ‘exploratory subgroup analysis’ is a more data-driven process that increasingly relies on the use of machine learning techniques (such as the use of unsupervised clustering algorithms) to discover new subgroups based on the analysis of a very large number of covariates (i.e., demographic, genomic, clinical, or other patient characteristics) and their impact on treatment response. This is an important process, not only for identifying which ‘patients’ may benefit the most from a specific drug, but also for the prevention of overfitting and bias, and making the estimates of treatment effect size more ‘honest’.
There is no one agreed method for conducting post-hoc exploratory subgroup analysis, and whilst there are guidelines available to guide the process of prospective confirmatory subgroup analysis no such guidelines yet exist for data-driven subgroup discovery. It is likely that such guidelines will develop over time, particularly as the drive towards personalised or precision medicine gathers pace. In the meantime, it is important that those conducting post-hoc analysis, particularly those using machine learning techniques, document the process, pay attention to basic best practice guidelines for statistical analysis (e.g., how to avoid p-hacking), and consider any appropriate responsible AI guidelines.
An Owkin example
In June 2023, Owkin published a paper in Nature Communications describing PACpAInt, a new histology-based deep learning model to decode the complexity of pancreatic cancer. For better patient prognosis and treatment, it’s vital to diagnose the molecular subtype of a patient’s tumor. PACpAInt compared survival distributions between different populations, clearly identifying two subgroups - one group of clearly differentiated tumors and one where the unclear tumors showed no clear pattern of classic or basal features.
It also uncovered a new subgroup of patients - a third of tumors analyzed were a mix of both classic and basal features - and found that patients with these mixed tumors were predicted to have a different prognosis. Finally, PACpAInt was also able to subtype patients based on specific non-cancer cells within the tumor - which opens up new possibilities for patient stratification in drug targeting trials. By providing a tool that’s easy to implement and could potentially decide treatment instantly without lengthy and costly RNA sequencing analysis, PACpAInt opens the way for patient stratification based on powerful molecular criteria.
Further reading
- Ballarini, Nicolás M. et al. 2018. ‘Subgroup Identification in Clinical Trials via the Predicted Individual Treatment Effect’ ed. Alan Hubbard. PLOS ONE 13(10): e0205971.
- Bunouf, Pierre, Mélanie Groc, Alex Dmitrienko, and Ilya Lipkovich. 2022. ‘Data-Driven Subgroup Identification in Confirmatory Clinical Trials’. Therapeutic Innovation & Regulatory Science 56(1): 65–75.
- Burke, James F, Jeremy B Sussman, David M Kent, and Rodney A Hayward. 2015. ‘Three Simple Rules to Ensure Reasonably Credible Subgroup Analyses’. BMJ: h5651.
- Lipkovich, Ilya, Alex Dmitrienko, and Ralph B. 2017. ‘Tutorial in Biostatistics: Data-Driven Subgroup Identification and Analysis in Clinical Trials: I. LIPKOVICH, A. DMITRIENKO AND R. B. D’AGOSTINO, SR.’ Statistics in Medicine36(1): 136–96.
- Loh, Wei‐Yin, Luxi Cao, and Peigen Zhou. 2019. ‘Subgroup Identification for Precision Medicine: A Comparative Review of 13 Methods’. WIREs Data Mining and Knowledge Discovery 9(5). https://onlinelibrary.wiley.com/doi/10.1002/widm.1326 (August 8, 2023).
- Zhang, Zhongheng et al. 2018. ‘Subgroup Identification in Clinical Trials: An Overview of Available Methods and Their Implementations with R’. Annals of Translational Medicine 6(7): 122–122.