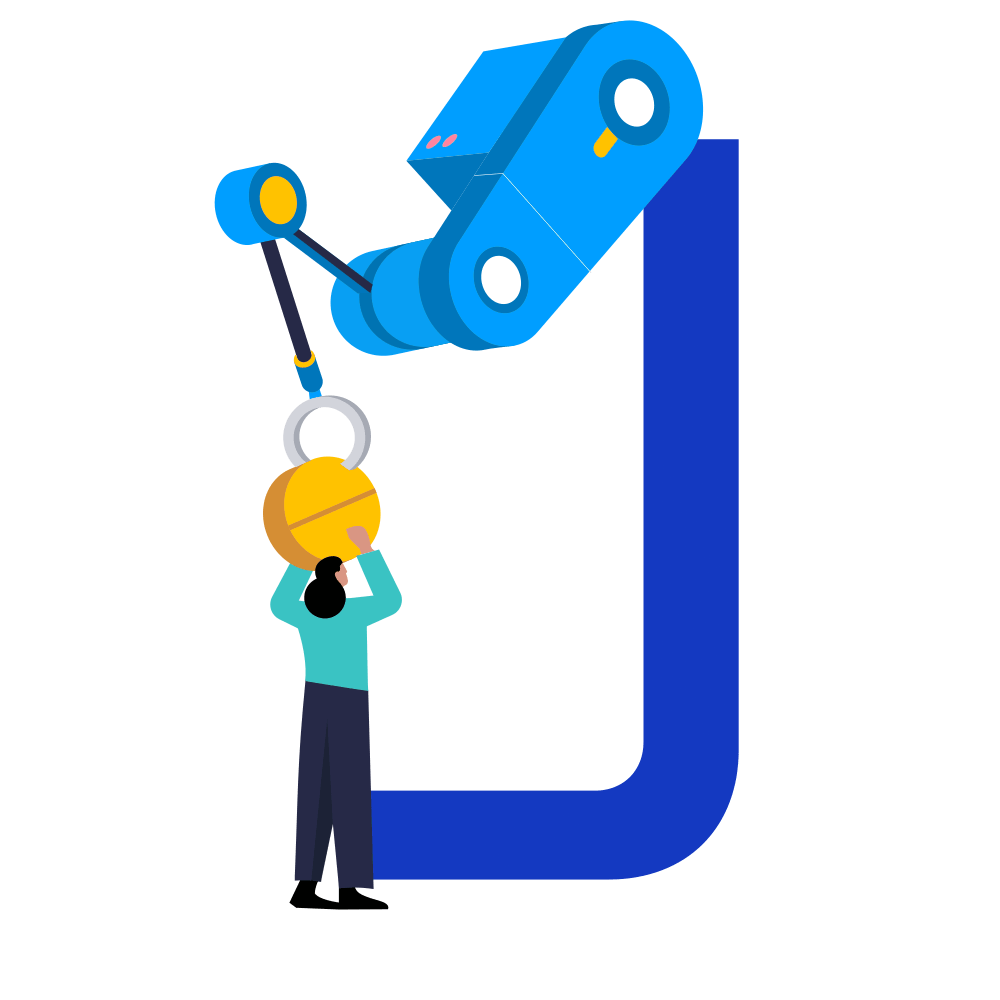
Job Enhancement
Job enhancement is a strategy used to improve job satisfaction and motivation by improving efficiency and adding more meaning and challenge to existing jobs.
Despite popular headlines such as ‘the algorithm will see you now’ giving the impression that AI will replace clinicians, it is more likely that AI will enhance rather than detract from the role of human clinicians. This is because AI can help clinicians in several ways.
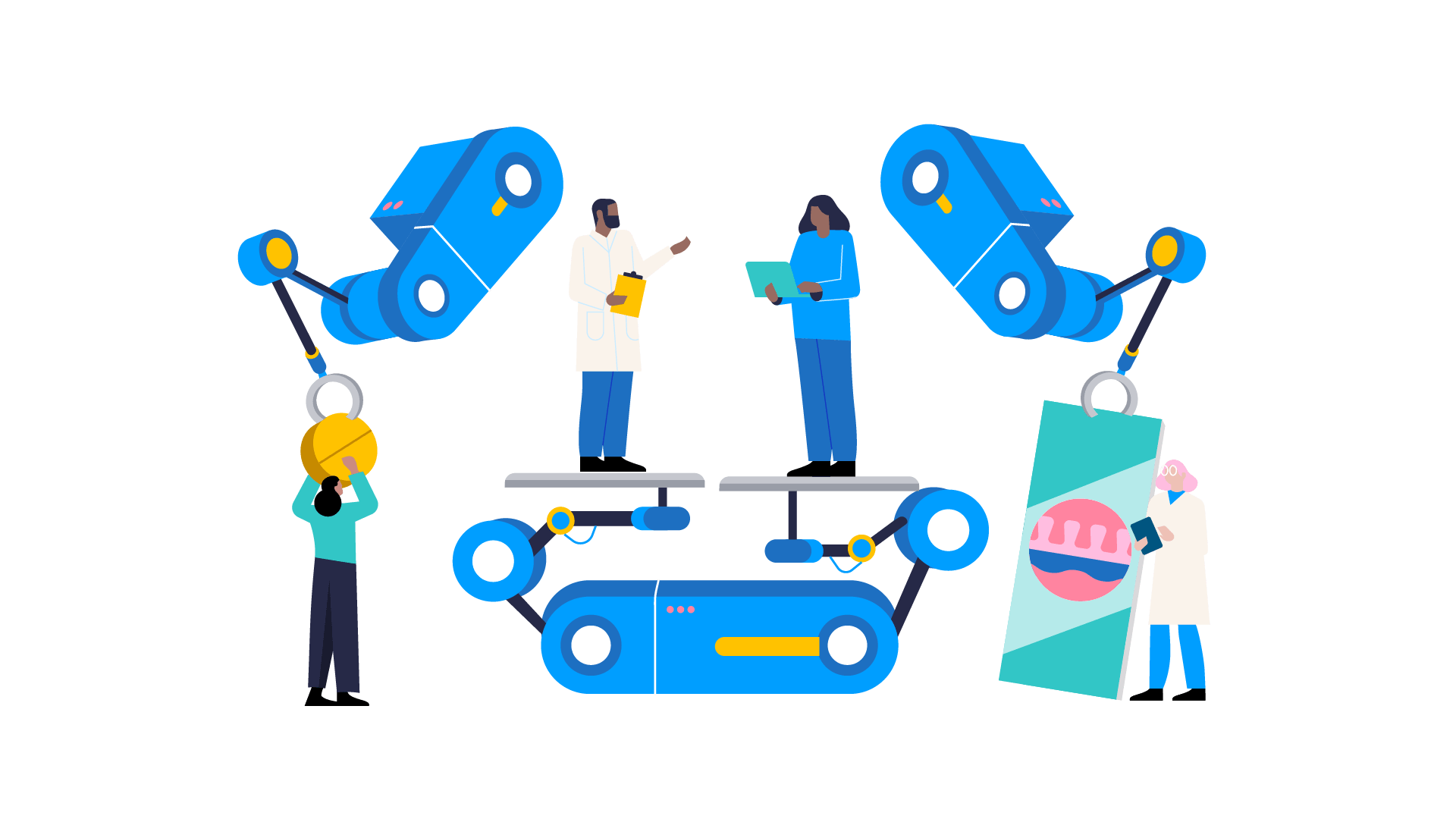
By helping with these kinds of activities, AI can make clinician jobs more efficient and less mundane, freeing up time to focus on the more interesting and challenging parts of the job such as creative problem solving and building relationships with patients. In this way, AI could help improve clinician job satisfaction and motivation and be a useful tool to include in any healthcare job enhancement strategy.
The concept of job enhancement (or enrichment) was first developed by Frederick Hertzberg in the 1950s as part of his two-factor theory. According to this theory, employee behaviour is determined by ‘hygiene factors’ and ‘motivating factors.’
Low job dissatisfaction and high motivation results in high employee performance and has benefits for retention. Successful job enhancement strategies focus on improving satisfaction and motivation by, for example, increasing job skill, job meaning, creating autonomy, providing better feedback, and making work more efficient.
Although AI is often described as being a ‘replacement’ for clinicians in the popular media, it is very unlikely that AI could ever genuinely fully replace the role of human clinicians. Healthcare is a fundamentally human activity that relies heavily on uniquely human traits such as empathy that can never meaningfully be replicated by AI.
Instead, it is more likely that AI will augment the role of human clinicians by automating mundane and repetitive tasks such as transcribing clinical notes or scheduling appointments. In doing so, AI can make the provision of healthcare more efficient, reducing the administrative burden placed on clinicians and releasing their time so that they can focus on the more complex and highly-skilled aspects of their job such as creative problem solving.
In addition, AI can help provide clinicians with real-time feedback on their performance by monitoring the decisions they make and comparing these decisions to those made by comparable clinicians, offer support, and help identify knowledge gaps that could be filled with additional training. All of this ‘help’ may ultimately improve job satisfaction and motivation, which is why AI can be a useful tool to include in any job enhancement strategy.
It is, however, worth noting that the introduction of AI will not automatically have a positive impact on satisfaction and motivation – indeed, it could undermine both – it all depends on how the AI is implemented. Thus, any job enhancement strategies including an AI element must pay careful attention to the implementation plans.
An Owkin example
Owkin is working to deliver AI diagnostics that integrate seamlessly into the digital pathology workflow to support accurate diagnosis at a fraction of the time and cost of existing tests. Our solutions help potentiate pathology workflows, pre-screen for biomarkers, and predict outcomes— giving healthcare providers a fuller picture of a patient’s disease. This means more patients can benefit from targeted therapies, making precision medicine more accessible to more patients at an earlier stage of their disease.
One of these solutions is MSIntuit CRC, a recently validated AI diagnostic which optimizes the testing procedure for microsatellite instability (MSI) in colorectal cancer. Using deep learning technology, it unprecedentedly leverages information contained on H&E slides to detect microsatellite stability (MSS) phenotypes with equivalent sensitivity compared to standard testing techniques.
Through ruling out those patients who would not benefit from immunotherapy during H&E slide diagnosis, MSIntuit CRC introduces a pre-screening step which saves valuable human time and resources - especially important considering the global shortage of pathologists. Such an optimization supports the scaling of MSI screening for more patient populations and further disease indications.
Further reading
- Crigger, Elliott, and Christopher Khoury. 2019. ‘Making Policy on Augmented Intelligence in Health Care’. AMA Journal of Ethics 21(2): E188-191.
- Crigger, Elliott et al. 2022. ‘Trustworthy Augmented Intelligence in Health Care’. Journal of Medical Systems 46(2): 12.
- Hazarika, Indrajit. 2020. ‘Artificial Intelligence: Opportunities and Implications for the Health Workforce’. International Health 12(4): 241–45.
- Li, Chao et al. 2023. ‘Does Artificial Intelligence Promote or Inhibit On-the-Job Learning? Human Reactions to AI at Work’. Systems 11(3): 114.
- Tursunbayeva, Aizhan, and Maarten Renkema. 2022. ‘Artificial Intelligence in Health‐care: Implications for the Job Design of Healthcare Professionals’. Asia Pacific Journal of Human Resources: 1744-7941.12325.
- The White House. 2022. The impact of Artificial Intelligence on the Future of Workforces in the European Union and the United States of America: An Economic Study Prepared in Response to the US-EU Trade and Technology Council Inaugural Joint Statement. The White House. https://www.whitehouse.gov/cea/written-materials/2022/12/05/the-impact-of-artificial-intelligence/